Simulated Data Models & the Future of AI for Mechanical Engineers
- Kai Thomas
- Jul 31, 2023
- 6 min read
Updated: Aug 29, 2023
After a discussion with one of our customers from the mechanical engineering industry on the topic of know-how sharing, today we would like to go a little deeper into the topic of "Simulated Data Models and the Future of AI for Mechanical Engineers" and gladly share some of our knowledge. If there are any deeper questions, feel free to send us a PM.
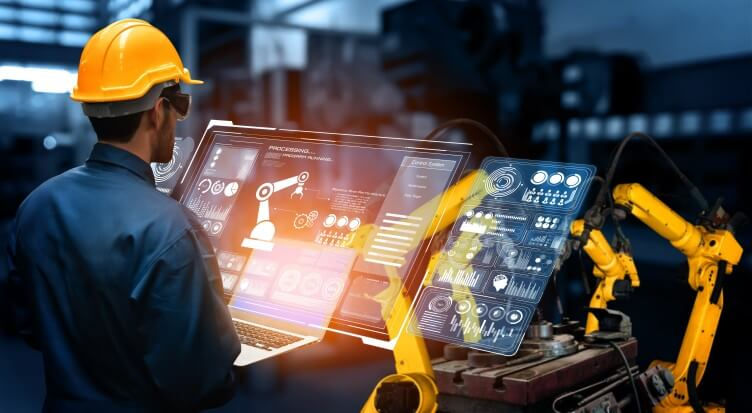
What are simulated data models?
First, a brief explanation of what simulated data models are in the first place:
Simply put, they are a form of generative artificial intelligence (AI) that are trained on large datasets of real-world data. These models can then be used to generate new data that is similar to the original data. This can be used for a variety of tasks, including natural language generation, image synthesis, and data augmentation for training other AI systems.
In the field of mechanical engineering, simulated data models can be used for a variety of applications, e.g:
Design and test new products: Simulated data models can simulate the performance of new products under various conditions. This can help engineers identify potential problems in the design and make necessary changes before the product is manufactured. In addition, simulated data models also allow prototypes to be tested in virtual environments, reducing the need for physical prototypes and saving development costs (topic "Digital Twin". There will be a separate article on this in the coming weeks). This advanced method accelerates the innovation process and enables companies to bring products to market faster and more cost-effectively.
Optimization of manufacturing processes: Simulated data models can be used to optimize manufacturing processes. This helps to reduce costs, improve quality and increase productivity. In conjunction with IoT models and products, the data models can also be reliably re-validated by collecting real-time data from the machines and devices. This, in turn, allows for early detection of potential malfunctions or failures, leading to efficient predictive maintenance. The combination of simulated data models, IoT and predictive maintenance makes it possible to continuously optimize manufacturing processes and ensure smooth production.
Diagnosis and troubleshooting of problems: Simulated data models can be used to diagnose and troubleshoot problems with existing products. In addition, simulated data models also enable the identification of potential vulnerabilities in existing products and systems, leading to productive maintenance and minimizing unforeseen failures. All of this helps to identify problem causes more quickly, develop problem solutions faster, extend the life of products, and ultimately significantly increase customer satisfaction.
Benefits of simulated data models
The potential benefits of simulated data models for mechanical engineers are enormous: the models will help engineers to accelerate the design, manufacture and operation of products, making them more sustainable. This leads to increased efficiency, productivity and profitability.
In addition to the benefits mentioned above, simulated data models can also be used to:
... generate new ideas and concepts: Simulated data models are ideal for developing new ideas and concepts for products and help engineers think outside the box to find innovative solutions to challenges faster.
Speed of idea generation: Generative AI can produce numerous design variants in a short time. By analyzing large amounts of data and patterns, it can produce innovative concepts that might not otherwise have been considered. This speed greatly improves the idea generation process and saves valuable time in the product development phase.
Design Optimization: Through iterative processes, simulated data models continuously refine and improve the design based on the desired performance criteria. This streamlines the optimization process, resulting in more efficient and optimal concepts in less time.
Exploring "unexplored" areas: Simulated data models explore unconventional design spaces and break away from traditional approaches as they do not have to deal with the "emotional experience" factor. This opens up opportunities for new and breakthrough ideas and fosters creativity and innovation in mechanical engineering.
Data-driven decision making: AI's ability to analyze massive amounts of data enables engineers to make informed decisions based on real-world performance insights. This data-driven approach ensures that design decisions are based on empirical evidence rather than intuition alone.
Resource efficiency: By reducing the number of physical prototypes required, simulated data models minimize the material waste and costs associated with traditional trial-and-error design approaches. It streamlines the design process and allows engineers to focus on refining the most promising concepts.
Collaboration and iteration: Collaboration between team members is facilitated because generative AI provides a common starting point for discussion and evaluation. Engineers can iterate and fine-tune designs together, resulting in higher quality concepts and solutions.
In short, simulated data models help engineers explore a larger design space, leading to faster and more innovative concepts. The technology complements traditional design methods and can have a significant positive impact on the efficiency and effectiveness of the product development process.
... train and educate employees: Simulated data models can be used to educate and train engineers because they provide a realistic and dynamic learning environment. This helps to improve the skills and knowledge of engineers and employees and prepare them for the challenges of the future. Especially here, other technologies such as virtual and augmented reality can be combined in a very useful way. (Topic "AR, VR, MR in mechanical engineering". There will be a separate article on this in the coming weeks).
Real-world experience: Simulated data models replicate real-world scenarios, allowing engineers to gain hands-on experience without the need for physical devices or prototypes. This hands-on experience promotes a deeper understanding of complex concepts and improves problem-solving skills.
Risk-free learning: Engineers can experiment and test different approaches in a risk-free environment. Mistakes made in simulations have no real-world consequences, so learners can learn from their mistakes without any negative impact on safety or cost.
Multidimensional analysis: Simulated data models provide the ability to analyze complex systems from multiple perspectives, giving engineers a comprehensive understanding of the various factors that influence their ideas and designs.
Interactive learning: Simulated data models promote interactive learning and allow engineers to explore the impact of design changes in real time. This interactive nature has been shown to promote engagement and knowledge retention.
Cost and time efficiency: Training with simulated data models eliminates the need for expensive physical prototypes and equipment, which significantly reduces training costs. In addition, learners can access the simulations whenever they want, saving time and resources.
Collaborative training: Simulated data models facilitate collaborative learning among engineering teams. Engineers can work together on projects, share ideas, and receive feedback, which promotes teamwork and communication skills.
Lifelong learning: Simulated data models provide continuous learning opportunities throughout a career. Engineers can keep up to date with the latest technologies and best practices, ensuring continuous professional development.
Simulated data models offer a safe, efficient and effective way to educate and train employees, especially engineers, and provide them with the necessary skills and experience to further their careers. Ultimately, this also makes the respective company more successful through constant innovation and even more attractive to other employees in times of a shortage of skilled workers.
Future of simulated data models
The future of simulated data models in mechanical engineering is promising and certainly has the potential to revolutionize the industry. The more sophisticated the models become, the more valuable they will be to engineers. The data models will help design, manufacture, operate and maintain products more efficiently and effectively. The ability to develop new ideas and concepts for products will also play a big role. Mechanical engineering companies should quickly pick up speed here, so that Made-in-Germany does not soon become a synonym for the "extended workbench" of other nations.
However, it is important to also be aware of potential risks associated with simulated data models. These models can produce data that is not representative of the real world. This sometimes leads to inaccurate results and misleading conclusions. In general, it is still important to use simulated data models with caution and always validate the results against real data.
And what does all this have to do with metavers GmbH?
We are an innovation agency that, in addition to the automotive industry, has also been advising companies from the mechanical engineering sector since the very beginning, developing future and productization strategies and their implementation, including digital products.
Since 2012, we have been working with Big Data and Deep Learning models, which formed and still form the basis for today's generative AI approaches.
Thereby, we are specialized in the following topics in the area of dealing with simulated data models:
Consulting and assistance in the general use of simulated data models (incl. workshops & hackathons).
Support in accessing the necessary data and resources for the use of simulated data models
Training on the efficient use of simulated data models
Support for the development and validation of simulated data models
Support in integrating simulated data models into their existing workflows
We are happy to support you in accelerating your own digital transformation through the use of simulated data models. By providing the above services, we help mechanical engineering companies to get the most out of simulated data models and to use this technology in a targeted and focused strategic manner.